Generative AI is revolutionizing the way we listen to the “voice” of the customer, whether during interactions in contact centers, in satisfaction surveys or on social networks. Thanks to an exhaustive analysis of written or vocal verbatims, combined with the “expertise” inherent in their LLM, these AIs promise to accurately answer complex questions and identify new topics.
The limits of traditional speech and text analytics
Customers express themselves through multiple written or vocal channels – interactions with the contact center, satisfaction surveys and social networks. These verbatims constitute a mine of information that can be exploited live or recorded using text analytics or speech anaytics tools, which have been greatly improved and democratized in recent years. Thanks to NLP (Natural Language Processing) algorithms boosted by deep learning, they are able to identify words or groups of words that translate recurring topics. For example, quotes from competitors or irritants that increase the risk of churn (loss of a customer). However, this type of analysis reaches its limits in terms of finesse, complexity and relevance. Irritants are certainly highlighted, but to fully understand their causes, it is necessary to manually consult the verbatims attached to the subject. A general, imprecise analysis needs to be supplemented by a targeted analysis that allows us to go into more detail.
Generative AI revolutionizes hot and cold analysis
Capable of swallowing millions of written or vocal conversations, generative AIs based on LLMs promise to reconcile comprehensiveness and accuracy. In this way, they can answer complex questions, while at the same time providing summaries of verbatims justifying these answers. For example, where conventional NLP tools can identify competitors cited by customers, LLM explains in detail the strengths and weaknesses of these competitors in terms of pricing or quality of offer or service. What’s more, this analysis takes into account changes over time, and even breakthroughs, such as a competitor who has just announced a better-performing or cheaper product. On the other hand, whereas conventional NLP simply classifies previously identified contact patterns, generative AI detects the emergence of new patterns – implicitly present in the data on which the LLM has been trained. And when a satisfaction survey is used to calculate an NPS (Net Promoter Score), analysis of the survey verbatims reveals the reasons why customers would (or would not) recommend the brand as a whole, as well as specific products or services.
All this information can be returned in a variety of forms: reports for general management, sales arguments for advisors, suggestions for new marketing actions, updates to a knowledge base. What’s more, the tool can be put into the hands of business users, who will express their queries in natural language and obtain relevant answers, also in natural language. Finally, the same generative AI can react in real time with the same precision, but this time at the level of each customer and each request, by triggering an alert or suggesting a response or marketing action.
In recent months, all Voice of the Customer (VoC) analysis tool vendors have been moving in this direction, albeit with varying degrees of maturity, particularly in terms of their ability to handle the French language, which makes the choice of a solution all the more critical.
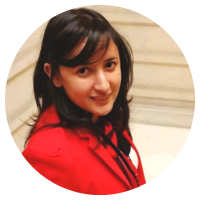
Fatiha Zelmat, CEO & Co-founder Ornidex